Note: Follow @ApacheAirflow on Twitter for the latest news and announcements!
We’ve just released Apache Airflow 2.4.1.
📦 PyPI: https://pypi.org/project/apache-airflow/2.4.1/
📚 Docs: https://airflow.apache.org/docs/apache-airflow/2.4.1
🛠️ Release Notes: https://airflow.apache.org/docs/apache-airflow/2.4.1/release_notes.html
🪶 Sources: https://airflow.apache.org/docs/apache-airflow/2.4.1/installation/installing-from-sources.html
Airflow PMC welcomes new Airflow Committer:
and new Airflow PMC members:
We’ve just released Apache Airflow 2.4.0. You can read all about what’s new in Apache Airflow 2.4.0 blog post.
📦 PyPI: https://pypi.org/project/apache-airflow/2.4.0/
📚 Docs: https://airflow.apache.org/docs/apache-airflow/2.4.0
🛠️ Release Notes: https://airflow.apache.org/docs/apache-airflow/2.4.0/release_notes.html
🪶 Sources: https://airflow.apache.org/docs/apache-airflow/2.4.0/installation/installing-from-sources.html
We’ve just released Apache Airflow 2.3.4.
📦 PyPI: https://pypi.org/project/apache-airflow/2.3.4/
📚 Docs: https://airflow.apache.org/docs/apache-airflow/2.3.4
🛠️ Release Notes: https://airflow.apache.org/docs/apache-airflow/2.3.4/release_notes.html
🪶 Sources: https://airflow.apache.org/docs/apache-airflow/2.3.4/installation/installing-from-sources.html
We’ve just released Apache Airflow 2.3.3.
📦 PyPI: https://pypi.org/project/apache-airflow/2.3.3/
📚 Docs: https://airflow.apache.org/docs/apache-airflow/2.3.3
🛠️ Release Notes: https://airflow.apache.org/docs/apache-airflow/2.3.3/release_notes.html
🪶 Sources: https://airflow.apache.org/docs/apache-airflow/2.3.3/installation/installing-from-sources.html
We’ve just released Apache Airflow 2.3.2.
📦 PyPI: https://pypi.org/project/apache-airflow/2.3.2/
📚 Docs: https://airflow.apache.org/docs/apache-airflow/2.3.2
🛠️ Release Notes: https://airflow.apache.org/docs/apache-airflow/2.3.2/release_notes.html
🪶 Sources: https://airflow.apache.org/docs/apache-airflow/2.3.2/installation/installing-from-sources.html
We’ve just released Apache Airflow 2.3.1.
📦 PyPI: https://pypi.org/project/apache-airflow/2.3.1/
📚 Docs: https://airflow.apache.org/docs/apache-airflow/2.3.1
🛠️ Release Notes: https://airflow.apache.org/docs/apache-airflow/2.3.1/release_notes.html
🪶 Sources: https://airflow.apache.org/docs/apache-airflow/2.3.1/installation/installing-from-sources.html
We’ve just released Apache Airflow Helm chart 1.6.0.
📦 ArtifactHub: https://artifacthub.io/packages/helm/apache-airflow/airflow
📚 Docs: https://airflow.apache.org/docs/helm-chart/1.6.0/
🛠️ Release Notes: https://airflow.apache.org/docs/helm-chart/1.6.0/release_notes.html
🪶 Sources: https://airflow.apache.org/docs/helm-chart/1.6.0/installing-helm-chart-from-sources.html
We’ve just released Apache Airflow 2.3.0. You can read more in the What’s new in Apache Airflow 2.3.0 blog post.
📦 PyPI: https://pypi.org/project/apache-airflow/2.3.0/
📚 Docs: https://airflow.apache.org/docs/apache-airflow/2.3.0
🛠️ Release Notes: https://airflow.apache.org/docs/apache-airflow/2.3.0/release_notes.html
🪶 Sources: https://airflow.apache.org/docs/apache-airflow/2.3.0/installation/installing-from-sources.html
We’ve just released Apache Airflow 2.2.5.
📦 PyPI: https://pypi.org/project/apache-airflow/2.2.5/
📚 Docs: https://airflow.apache.org/docs/apache-airflow/2.2.5/
🛠️ Changelog: Airflow 2019 Download 🪶 Sources: https://airflow.apache.org/docs/apache-airflow/2.2.5/installation/installing-from-sources.html
We’ve just released Apache Airflow Helm chart 1.5.0.
📦 ArtifactHub: https://artifacthub.io/packages/helm/apache-airflow/airflow
📚 Docs: https://airflow.apache.org/docs/helm-chart/1.5.0/
🛠️ Changelog: https://airflow.apache.org/docs/helm-chart/1.5.0/changelog.html
🪶 Sources: https://airflow.apache.org/docs/helm-chart/1.5.0/installing-helm-chart-from-sources.html
Airflow Summit 2022
The biggest Airflow Event of the Year returns May 23–27! Airflow Summit 2022 will bring together the global community of Apache Airflow practitioners and data leaders.
What’s on the Agenda
During the free conference, you Airflow 2019 Download hear about Apache Airflow best practices, trends in building data pipelines, data governance, Airflow and machine learning, and the future of Airflow. There will also be a series of presentations on non-code contributions driving the open-source project.
How to Attend
This year’s edition will include a variety of online Airflow 2019 Download across different time zones. Additionally, Airflow 2019 Download, you can take part in local in-person events organized worldwide for data communities to watch the event and network.
Interested?
🪶 Register for Airflow Summit 2022 today!
🗣️ If you have an Airflow story to share, join as a speaker
✨ Follow Airflow Summit on LinkedIn to stay current with the latest updates.
🤝 Check out the in-person events planned for Airflow Summit 2022.
We’ve just released Apache Airflow 2.2.4.
📦 PyPI: https://pypi.org/project/apache-airflow/2.2.4/
📚 Docs: https://airflow.apache.org/docs/apache-airflow/2.2.4/
🛠️ Changelog: https://airflow.apache.org/docs/apache-airflow/2.2.4/changelog.html
🪶 Sources: https://airflow.apache.org/docs/apache-airflow/2.2.4/installation/installing-from-sources.html
Airflow PMC welcomes two new Airlow Committers:
We’ve just released Apache Airflow Helm chart 1.4.0.
📦 ArtifactHub: https://artifacthub.io/packages/helm/apache-airflow/airflow
📚 Docs: https://airflow.apache.org/docs/helm-chart/1.4.0/
🛠️ Changelog: https://airflow.apache.org/docs/helm-chart/1.4.0/changelog.html
🪶 Sources: https://airflow.apache.org/docs/helm-chart/1.4.0/installing-helm-chart-from-sources.html
Airflow PMC welcomes Jed Cunningham (@jedcunningham) as the newest addition to Airflow PMC.
We’ve just released Apache Airflow 2.2.3.
📦 PyPI: https://pypi.org/project/apache-airflow/2.2.3/
📚 Docs: https://airflow.apache.org/docs/apache-airflow/2.2.3/
🛠️ Changelog: https://airflow.apache.org/docs/apache-airflow/2.2.3/changelog.html
🪶 Sources: https://airflow.apache.org/docs/apache-airflow/2.2.3/installation/installing-from-sources.html
We’ve just released Apache Airflow 2.2.2.
📦 PyPI: https://pypi.org/project/apache-airflow/2.2.2/
📚 Docs: https://airflow.apache.org/docs/apache-airflow/2.2.2/
🛠️ Changelog: https://airflow.apache.org/docs/apache-airflow/2.2.2/changelog.html
🪶 Sources: https://airflow.apache.org/docs/apache-airflow/2.2.2/installation/installing-from-sources.html
We’ve just released Apache Airflow Helm chart 1.3.0.
📦 ArtifactHub: https://artifacthub.io/packages/helm/apache-airflow/airflow
📚 Docs: https://airflow.apache.org/docs/helm-chart/1.3.0/
🛠️ Changelog: https://airflow.apache.org/docs/helm-chart/1.3.0/changelog.html
🪶 Sources: https://airflow.apache.org/docs/helm-chart/1.3.0/installing-helm-chart-from-sources.html
We’ve just released Apache Airflow 2.2.1.
📦 PyPI: https://pypi.org/project/apache-airflow/2.2.1/
📚 Docs: https://airflow.apache.org/docs/apache-airflow/2.2.1/
🛠️ Changelog: https://airflow.apache.org/docs/apache-airflow/2.2.1/changelog.html
🪶 Sources: https://airflow.apache.org/docs/apache-airflow/2.2.1/installation/installing-from-sources.html
We’ve just released Apache Airflow 2.2.0. You can read more in the What’s new in Apache Airflow 2.2.0 blog post.
📦 PyPI: https://pypi.org/project/apache-airflow/2.2.0/
📚 Docs: https://airflow.apache.org/docs/apache-airflow/2.2.0/
🛠️ Changelog: https://airflow.apache.org/docs/apache-airflow/2.2.0/changelog.html
🪶 Sources: https://airflow.apache.org/docs/apache-airflow/2.2.0/installation/installing-from-sources.html
We’ve just released Apache Airflow Helm chart 1.2.0.
📦 ArtifactHub: https://artifacthub.io/packages/helm/apache-airflow/airflow
📚 Docs: https://airflow.apache.org/docs/helm-chart/1.2.0/
🛠️ Changelog: https://airflow.apache.org/docs/helm-chart/1.2.0/changelog.html
🪶 Sources: https://airflow.apache.org/docs/helm-chart/1.2.0/installing-helm-chart-from-sources.html
We’ve just released Apache Airflow 2.1.4.
📦 PyPI: https://pypi.org/project/apache-airflow/2.1.4/
📚 Docs: https://airflow.apache.org/docs/apache-airflow/2.1.4/
🛠️ Changelog: https://airflow.apache.org/docs/apache-airflow/2.1.4/changelog.html
🪶 Sources: https://airflow.apache.org/docs/apache-airflow/2.1.4/installation/installing-from-sources.html
Airflow PMC welcomes 2 new members to the Airflow PMC:
Airflow PMC welcomes Brent Bovenzi (@bbovenzi) as the newest Airflow Committer. 👏👏
We’ve just released Apache Airflow 2.1.3.
📦 PyPI: https://pypi.org/project/apache-airflow/2.1.3/
📚 Docs: https://airflow.apache.org/docs/apache-airflow/2.1.3/
🛠️ Changelog: https://airflow.apache.org/docs/apache-airflow/2.1.3/changelog.html
🪶 Sources: https://airflow.apache.org/docs/apache-airflow/2.1.3/installation.html#installing-airflow-from-released-sources-and-packages
We’ve just released Apache Airflow 2.1.2.
📦 PyPI: https://pypi.org/project/apache-airflow/2.1.2
📚 Docs: https://airflow.apache.org/docs/apache-airflow/2.1.2/
🛠️ Changelog: https://airflow.apache.org/docs/apache-airflow/2.1.2/changelog.html
🪶 Sources: https://airflow.apache.org/docs/apache-airflow/2.1.2/installation.html#installing-airflow-from-released-sources-and-packages
Airflow PMC welcomes Aneesh Joseph (@aneesh-joseph) as the newest Airflow Committer. 👏👏
We’ve just released Apache Airflow 2.1.1.
📦 PyPI: https://pypi.org/project/apache-airflow/2.1.1
📚 Docs: https://airflow.apache.org/docs/apache-airflow/2.1.1/
🛠️ Changelog: https://airflow.apache.org/docs/apache-airflow/2.1.1/changelog.html
🪶 Sources: https://airflow.apache.org/docs/apache-airflow/2.1.1/installation.html#installing-airflow-from-released-sources-and-packages
Airflow PMC welcomes 2 new committers:
I’m happy to announce that Apache Airflow 2.1.0 was just released. This one includes a raft of fixes and other small improvements, but some notable additions include:
A Create a DAG Calendar View to show the status of your DAG run across time more easily
The cross-dag-dependencies view (which used to be an external plugin) is now part of core
Mask passwords and sensitive info in task logs and UI (finally!)
Improvmenets to webserver start up time (mostly around time spent syncing DAG permissions)
Please note that this release no long Airflow 2019 Download the extra provider by default, as we discovered that it pulls in an LGPL dependency (via the module of all places) so it is now optional.
📦 PyPI: https://pypi.org/project/apache-airflow/2.1.0
📚 Docs: https://airflow.apache.org/docs/apache-airflow/2.1.0/
🛠️ Changelog: https://airflow.apache.org/docs/apache-airflow/2.1.0/changelog.html
🪶 Sources: https://airflow.apache.org/docs/apache-airflow/2.1.0/installation.html#installing-airflow-from-released-sources-and-packages
The first release of our official helm chart for Apache Airflow is here!
📦 ArtifactHub: https://artifacthub.io/packages/helm/apache-airflow/airflow
📚 Docs: https://airflow.apache.org/docs/helm-chart/1.0.
🚀 Quick Start Installation Guide: https://airflow.apache.org/docs/helm-chart/1.0.0/quick-start.html
Airflow PMC welcomes 2 new committers:
Airflow Summit will be held online July 8-16, 2021. To register or propose a talk go to official Airflow Summit website.
We’ve just released Airflow Backport Provider Packages 2020.10.5.
We’ve just released Apache Airflow 1.10.15.
📦 PyPI: https://pypi.org/project/apache-airflow/1.10.15
📚 Docs: https://airflow.apache.org/docs/apache-airflow/1.10.15/
🛠️ Changelog: https://airflow.apache.org/docs/apache-airflow/1.10.15/changelog.html
19 bug fixes, 3 Improvements & a couple of doc updates since 1.10.14
Apache Airflow Elasticsearch Provider 1.0.3 released
📦 PyPI: https://pypi.org/project/apache-airflow-providers-elasticsearch/1.0.3/
🛠️ Changelog: https://airflow.apache.org/docs/apache-airflow-providers-elasticsearch/1.0.3/index.html#changelog
We’ve just released Apache Airflow Upgrade Check 1.3.0:
📦 PyPI: https://pypi.org/project/apache-airflow-upgrade-check/1.3.0/
🛠️ Changelog: https://github.com/apache/airflow/tree/upgrade-check/1.3.0/airflow/upgrade#changelog
This powers the command to make upgrading to Apache Airflow 2.0 easier.
Airflow PMC welcomes 3 new committers:
Airflow PMC welcomes Ephraim Anierobi (@ephraimbuddy) as the newest Airflow committer.
We’ve just released Apache Airflow 2.0.1.
📦 PyPI: https://pypi.org/project/apache-airflow/2.0.1
📚 Docs: https://airflow.apache.org/docs/apache-airflow/2.0.1/
🛠️ Changelog: https://airflow.apache.org/docs/apache-airflow/2.0.1/changelog.html
We also released 61 updated and 2 new providers.
Airflow PMC welcomes Vikram Koka (@vikramkoka) as the newest Airflow Committer.
Airflow PMC welcomes Xiaodong Deng (@XD-DENG) as the newest addition to Airflow PMC.
Jeremiah Lowin has resigned from the Airflow PMC.
Thank you Jeremiah for all your contributions and involvement in Airflow’s early years.
We’ve just released Apache Airflow 2.0.0, Airflow 2019 Download. You can read more about what 2.0 brings in the announcement post.
📦 PyPI: https://pypi.org/project/apache-airflow/2.0.0
📚 Docs: https://airflow.apache.org/docs/apache-airflow/2.0.0/
We’ve just released Apache Airflow 1.10.14. This is a “bridge” release for Airflow 2.0.
PyPI: https://pypi.org/project/apache-airflow/1.10.14
Docs: https://airflow.readthedocs.io/en/1.10.14/
Changelog: https://airflow.readthedocs.io/en/1.10.14/changelog.html
We’ve just released Apache Airflow 1.10.13
PyPI: https://pypi.org/project/apache-airflow/1.10.13
Docs: https://airflow.readthedocs.io/en/1.10.13/
Changelog: https://airflow.readthedocs.io/en/1.10.13/changelog.html
405 commits since 1.10.12 (6 New Features, 31 Airflow 2019 Download, 30 Bug Fixes, and tons of doc changes and internal changes)
We’ve just released Airflow Backport Provider Packages 2020.10.5.
Airflow PMC welcomes Ryan Hamilton (@ryanahamilton) as the new Airflow Committer.
We’ve just released Airflow Backport Provider Packages 2020.10.5.
57 packages have been released.
We’ve just released Airflow v1.10.12
PyPI - https://pypi.org/project/apache-airflow/1.10.12/
Docs - https://airflow.apache.org/docs/1.10.12/
ChangeLog - https://airflow.apache.org/docs/1.10.12/changelog.html
113 commits since 1.10.10 (5 New Features, 23 Improvements, 23 Bug Fixes, and 14 doc changes)
Airflow PMC welcomes Leah Cole (@leahecole) and Ry Walker (@ryw) as new Airflow Committers.
We’ve just released Airflow v1.10.11
PyPI - https://pypi.org/project/apache-airflow/1.10.11/
Docs - https://airflow.apache.org/docs/1.10.11/
ChangeLog - https://airflow.apache.org/docs/1.10.11/changelog.html
306 Airflow 2019 Download since 1.10.10 (12 New Features, 90 Improvements, 53 Bug Fixes, and several doc changes)
Airflow PMC welcomes Daniel Imberman (@dimberman), Tomek Turbaszek (@turbaszek), and Kamil Breguła (@mik-laj) as new PMC members, and QP Hou (@houqp) as a committer, Airflow 2019 Download. Congrats!
The (virtual) Airflow Summit has begun – you can watch along at airflowsummit.org
We’ve just released Airflow Backport Provider Packages 2020.6.24
The Backport provider packages make it possible to easily use Airflow 2.0 Operators, Hooks, Sensors, Secrets, Transfers in Airflow 1.10, Airflow 2019 Download. More stats below, Airflow 2019 Download, but the Backport Provider packages increase the number of easily-available integrations for Airflow 1.10 users by Airflow 2019 Download whopping 55%.
- We have 58 backport packages in total. 599 classes (Operators, Hooks, Transfers, Sensors, Secrets)
- We have 213 new (!) classes that have not been easily available to 1.10 users so far:
- Operators: 150
- Transfers: 12
- Sensors: 14
- Hooks: 37
- Secrets: 0
- We have 386 classes that were moved. Quite a number of those (hard to say exactly how many) got new features, options, parameters.
- Operators: 204
- Transfers: 36
- Sensors: 46
- Hooks: 96
- Secrets: 4
List of the backport provider packages:
- Amazon
- Apache HDFS
- Apache Hive
- Apache Livy
- Apache Pig
- Apache Pinot
- Apache Spark
- Apache Sqoop
- Azure
- Cassandra
- Celery
- Cloudant
- Databricks
- Datadog
- Dingding
- Discord
- Docker
- Druid
- Elasticsearch
- Email
- Exasol
- Facebook
- FTP
- Google
- GRPC
- Hashicorp
- HTTP
- IMAP
- JDBC
- Jenkins
- JIRA
- Mongo
- MsSQL
- MySQL
- ODBC
- OpenFAAS
- OpsGenie
- Oracle
- PagerDuty
- Postgres
- Presto
- Qubole
- Redis
- Salesforce
- Samba
- Segment
- SFTP
- Singularity
- Slack
- Snowflake
- Sqlite
- SSH
- Vertica
- Winrm
- Yandex
- Zendesk
We’ve just released Airflow v1.10.10
PyPI - https://pypi.org/project/apache-airflow/1.10.10/
Docs - https://airflow.apache.org/docs/1.10.10/
Changelog - https://airflow.apache.org/docs/1.10.10/changelog.html
199 commits since 1.10.9 (11 New Features, 43 Improvements, 44 Bug Fixes, and several doc changes)
Airflow PMC welcomes Jiajie Zhong (@zhongjiajie) as its new committer. Congrats!
and
Second Warsaw Airflow Meetup is happening next week and it will be an online event!
We’ve just released Airflow v1.10.8
PyPI - https://pypi.org/project/apache-airflow/1.10.8/
Docs - https://airflow.apache.org/docs/1.10.8/
Changelog - http://airflow.apache.org/docs/1.10.8/changelog.html#airflow-1-10-8-2020-01-07
160 commits since 1.10.7 (4 new features, 42 improvements, Airflow 2019 Download, 36 bug fixes, and several doc changes)
and
We’ve just released Airflow 1.10.9 (this one is a quick fix to work around the breaking release of Werkzeug 1.0)
PyPI - https://pypi.org/project/apache-airflow/1.10.9/
Docs - https://airflow.apache.org/docs/1.10.9/
2 commits since 1.10.8 :)
We’ve just released Airflow v1.10.7
PyPI - https://pypi.org/project/apache-airflow/1.10.7/
Docs - https://airflow.readthedocs.io/en/1.10.7/
Changelog - https://github.com/apache/airflow/blob/1.10.7/CHANGELOG.txt
217 commits since 1.10.6 including some Critical Bugfixes and Performance Improvements (17 new features, 57 improvements, Airflow 2019 Download, 52 bug fixes, and several doc changes)
Airflow PMC welcomes Tomasz Urbaszek (@turbaszek) as its new committer, Airflow 2019 Download. Congrats!
Airflow PMC welcomes Kengo Seki (@sekikn) to both its committer and PMC ranks. Congrats!
New website for Apache Airflow is live : https://airflow.apache.org
Same URL with more & better webpages
Airflow PMC has voted in & promoted Aizhamal Nurmamat kyzy (@Aizhamal) and Kevin Yang (@KevinYang21) to be a part of Airflow PMC.
We’ve just released ApacheAirflow v1.10.6
PyPI - https://pypi.org/project/apache-airflow/1.10.6/
Docs - https://airflow.apache.org/1.10.6/
168 commits since 1.10.5 (including 6 new features, 35 improvements, 38 bug fixes, and the usual swathe of doc fixes and CI improvements)
Airflow PMC has voted in & promoted Jarek Potiuk (@higrys) to be a PMC Member.
Jarek has been one of the most active community members and has spread the word about Airflow Well deserved Jarek, congratulations
What do you think of our new logo? #AirflowLogo#ApacheAirflow
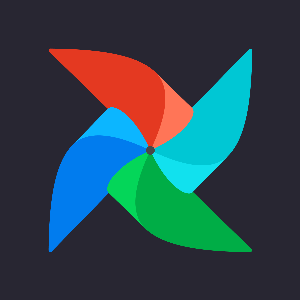
Two meetups are happening soon:
- Seattle, WA on Thursday 19th
- London, UK on Tuesday 24th
We’ll put links to any recordings here once they are available.
The PMC has just added two new Airflow Committers :
We’ve just released ApacheAirflow v1.10.5
PyPI - https://pypi.org/project/apache-airflow/1.10.5/
Docs - https://airflow.apache.org/1.10.5/
95 commits since 1.10.4 (including 3 new features, Airflow 2019 Download, 17 improvements, 23 bug fixes, and lots more doc fixes and CI improvements)
We’ve just released ApacheAirflow v1.10.4
PyPI - https://pypi.org/project/apache-airflow/1.10.4/
Docs - https://airflow.apache.org/1.10.4/
377 commits since 1.10.3 (including 50 new features, 107 improvements, 91 bug fixes, and lots more doc fixes and CI improvements)
The community welcomes the latest Apache Airflow Microsoft Office Crack 2021 + Product Key Latest Free group: The Melbourne Apache Airflow meetup. Stay tuned for its first meetup event!
The Apache Airflow PMC welcomes a slew of new committers to it ranks! The following contributors are now Airflow Committers:
Congratulations folks - Very well deserved.
New Apache Airflow release 1.10.3 is out!
Pypi - https://pypi.org/project/apache-airflow/1.10.3/ (Run )
Changelog - https://airflow.apache.org/1.10.3/changelog.html#airflow-1-10-3-2019-04-09
This release no longer needs the SLUGIFY_USES_TEXT_UNIDECODE/AIRFLOW_GPL_UNIDECODE environment variables at install time to avoid the GPL dependency!
The Apache Airflow NYC meetup is having its next meetup on April 29, 2019 – more details here.
If you are in or around NYC, please be sure to check it out!
The Apache Airflow PMC welcomes new committer, Daniel Imberman (@dimberman).
The Apache Airflow PMC welcomes new committer, Xiaodong Deng (XD-DENG)
We are pleased to announce our newest Meetup, this one in Portland, Oregon, USA.
New Apache Airflow release 1.10.2 is out!
Pypi - https://pypi.python.org/pypi/apache-airflow (Run )
Changelog - https://airflow.apache.org/changelog.html#airflow-1-10-2-2019-01-19
By default one of Airflow’s dependencies installs a GPL dependency (unidecode). To avoid this dependency set SLUGIFY_USES_TEXT_UNIDECODE=yes in your environment when you install or upgrade Airflow. To force installing the GPL version set AIRFLOW_GPL_UNIDECODE. One of these two environment variables must be specified.
The Apache Airflow Paris meetup is having its second meetup on Feb 6, 2019 – more details here.
If you are in or around Paris, please be sure to check it out!
Apache Airflow graduates from the Incubator and is now a TLP!
New Apache Airflow release 1.10.1-incubating is out!
Pypi - https://pypi.python.org/pypi/apache-airflow (Run )
Changelog - https://github.com/apache/incubator-airflow/blob/v1-10-test/CHANGELOG.txt
By default one of Airflow’s dependencies installs a GPL dependency (unidecode). To avoid this dependency set SLUGIFY_USES_TEXT_UNIDECODE=yes in your environment when you install or upgrade Airflow. To force installing the GPL version set AIRFLOW_GPL_UNIDECODE. One of these two environment variables must be specified.
We are excited to announce that the Paris Apache Airflow 2019 Download Meetup will be hosting its inaugural meetup on Nov 21
Speakers include Chauffeur Prive (event organizer). More to come soon!
The Singapore Big Data Meetup will host “Intro to Airflow” via @XD-DENG on Sept 27, 2018! Check it out! http://bit.ly/2PMgABT
We are excited to announce that the London Apache Airflow Meetup will be hosting its inaugural meetup on Sept 20.
Speakers include London committer Ash Berlin-Taylor & Ben Marengo from Just Eat!
The Bay Area Apache Airflow Meetup group will be hosting its next meetup at Google on Sept 24!.
Five 20-minute talks followed by 6 lightning sessions! Come one, come all!
We welcome the 7th & latest regional Apache Airflow Meetup, this one in Seattle.
The Apache Airflow Meetup group in Amsterdam will be hosting its 2nd meetup at GoDataDriven on Sep 12.
New Apache Airflow release 1.10.0-incubating is out!
Pypi - https://pypi.python.org/pypi/apache-airflow (Run )
Changelog - https://github.com/apache/incubator-airflow/blob/8100f1f/CHANGELOG.txt
By default one of Airflow’s dependencies installs a GPL dependency (unidecode). To avoid this dependency set SLUGIFY_USES_TEXT_UNIDECODE=yes in your environment when you install or upgrade Airflow. To force installing the GPL version set AIRFLOW_GPL_UNIDECODE. One of these two environment variables must be specified.
The Apache Airflow PPMC welcomes new committer and PPMC member, Tao Feng (@feng-tao)
We’re happy to welcome Airflow 2019 Download new Apache Airflow Meetup Ashampoo Audio Recorder Free Download – this one in London, UK
We’re happy to welcome a new Apache Airflow Meetup group – this one in Chicago.
The Apache Airflow PPMC welcomes new committer and PPMC member, Kaxil Naik (@Kaxil)
The Bay Area Apache Airflow Meetup group will be hosting a meetup on April 11 at WePay.
Speakers from Slack, Google, and WePay will be presenting.
The Apache Airflow PPMC welcomes new committer and PPMC member, Airflow 2019 Download, Ash Berlin-Taylor (@ashb)
New Apache Airflow release 1.9.0-incubating is out!
Pypi - https://pypi.python.org/pypi/apache-airflow (Run )
ReleaseNotes - https://github.com/apache/incubator-airflow/blob/master/CHANGELOG.txt
Special Thanks to Chris Riccomini (@criccomini) and Bolke utorrent pro download Bruin (@bolkedebruin) for tirelessly shepherding this release!
The Apache Airflow PPMC welcomes new committer and PPMC member, Joy Gao (@joygao)
The Apache Airflow Meetup group in Amsterdam will be hosting its 1st meetup (Heineken) on Dec 21.
Niels Zeilemaker from GoDataDriven will present Using Azure Container Instances as a cheap method to run Heineken data science workloads, and Daniel van der Ende from ING Wholesale banking Advanced Analytics will talk about Data Tests using Apache Airflow.
We'’re happy to welcome a new Apache Airflow Meetup group in Amsterdam
Next Bay Area Airflow meet-up hosted by Airbnb this December (Dec 4)
Slides are available here.
The Apache Airflow PPMC welcomes new committer and PPMC member, Fokko Driespong (@fokko)
New Apache Airflow release 1.8.2-incubating is out!
Pypi - https://pypi.python.org/pypi/apache-airflow
Release Notes - http://bit.ly/2gH9QFx
Special Thanks to Maxime Beauchemin (@mistercrunch) for tirelessly shepherding this release!
We'’re happy to welcome a new Apache Meetup group in Tokyo
Their first event is being held on May 11
Slides :
The first official Apache Airflow release (1.8.0-incubating) is out!
Git Tag:https://github.com/apache/incubator-airflow/releases/tag/1.8.0%2Bapache.incubating
Change log: 205 commits as shown in Changelog (1.8.0-incubating)
PyPi: coming soon, Airflow 2019 Download, check https://pypi.python.org/pypi/airflow
Note: remember to run after installation
Thanks to everyone in the community that Airflow 2019 Download bring this about. A special thanks is due to Bolke de Bruin (@bolkedebruin) for valiantly shepherding this release!
The Apache Airflow PPMC welcomes new committer and PPMC member, Alex Guziel (@saguziel)
We'’re happy Airflow 2019 Download welcome a new Apache Meetup group in NYC
Next Bay Area Apache Airflow Meet-up (at PayPal) on Mar 14 : http://bit.ly/2knyNHv
Next New York Apache Airflow Meet-up (at Blue Apron) on Feb 1
Sign-up:https://docs.google.com/spreadsheets/d/1WmfgZeExSVdLf-u1uh3IleeHy8QTwaJ4BkkSkVM-X1E/edit#gid=0
Time: 6:30 - 9pm EST
Location: 40 W 23rd St. New York, NY 10010 (5th floor)
The Apache Airflow PPMC welcomes new committer and PPMC member, Alex Van Boxel (@alexvanboxel)
Video from the Nov 16 meet-up (at WePay) is now available at : https://wepayinc.app.box.com/s/1183ra3z8gxf8fridysu4wbjckg1s05v
New Apache Airflow Meet-up (at WePay) on Nov 16 : http://bit.ly/2dwKNls
New Apache Airflow Meet-up (at Stripe) on Sept 21 : http://www.meetup.com/Bay-Area-Apache-Airflow-Incubating-Meetup/events/233316814/
New Warsaw (Poland) Hadoop User Group Meet-up on Sept 14 : http://www.meetup.com/warsaw-hug/events/233912332/
The Apache Airflow PPMC welcomes new committer and PPMC member, Li Xuanji (@zodiac))
The Apache Airflow PPMC welcomes new committer and PPMC member, Sumit Maheshwari (@msumit)
Video of yesterday’s Apache Airflow Meet-up talk featuring talks from WePay, Agari, and Yahoo is now available at http://bit.ly/1S69hOr
The first-ever Airflow Contributor Meeting will take place at 11AM PST on June 1st.
https://airbnb.webex.com/join/gurer.kiratli
Agenda
- Ways to mitigate risks when rolling out new Airflow releases into production
- Clarifying the process for working on large efforts
- Refreshing the current roadmap
- Communicate about how Airbnb will share information about sprints and internal roadmap moving forward
Airflow 1.7.1.2 released: Pypi or via Git tag – 214 commits as per CHANGELOG.txt
Special thanks to Dan Davydov (@aoen) for tirelessly shepherding this release!
Steven Yvinec-Kruyk (@syvineckruyk) joins the Apache Airflow Committer and PPMC group today. Please give him a hearty welcome and of course., Airflow 2019 Download. ask him to review your PRs and answer any questions you may have :)
Want to keep abreast of new Apache Airflow updates (e.g. releases, meet-ups, new features, conference talks, etc… ), Airflow 2019 Download, follow our newly-minted Twitter account Airflow 2019 Download @ApacheAirflow
We are starting the migration to Apache Infrastructure (e.g. GitHub Issues –> Jira, Airflow 2019 Download, Airbnb/Airflow GitHub to Apache/Airflow GitHub, Airbnb/Airflow GitHub Wiki to Apache Airflow Confluence Wiki)
- The progress and migration status will be tracked on Migrating to Airflow 2019 Download expect this to take roughly 1 week. On and after May 4, we expect to be using Apache infrastructure exclusively. To prepare for that day, start using the new Apache infrastructure and follow instructions on this JIRA ticket AIRFLOW-11 to set up your accounts.
Sid Anand (@r39132) will be speaking on July 23 at Data Day Seattle
- If there is enough interest, I’d be happy to also speak at a meetup
A 1.7.1. lightweight tag was mistakenly pushed to master - we don’t yet have a viable 1.7.1 release candidate. On April 12, @r39132 deleted the tag on master
We now have an Apache Airflow meet-up group for folks in the Bay Area: Sign up today to get notified of upcoming meet-ups!
Folks, A new release (1.7.0) is out via Pypi and git tag
Folks, A new release candidate version of Airflow is ready for the community to try: 1.7.0rc1
- Please check it out and report any issues that you see
This week, we applied for Airflow’s entry to the Apache Incubator
- Airflow Proposal
- We promoted several contributors to committers based on a proven track-record of contributions to the project and a strong commitment to improving the project going forward
- We published a WIP Roadmap [ARCHIVED] and welcome comments and input from the community
Airbnb will be hosting the very first Airflow meetup at Airbnb HQ (888 Brannan, in SF) on March 28th.
We’re planning on doing this regularly, and want to put a good line up of food, drinks and talks for you all.
It should be pretty informal, but here’s a draft of the schedule for the night:
- meet, geet and eat
- updates on the project, Airflow 2019 Download, roadmap & upcoming Airbnb sprints
- present advanced/complex Airbnb Airflow use cases (A/B testing framework, anomaly detection or something of that nature)
- Sid Anand will share about how they use Airflow at Agari
- Q/A for the core project team
- Community open mic, Airflow 2019 Download, step up and make announcements if you’re recruiting, looking for help, planning on working on a feature, …
- Define list of subjects of interest on a whiteboard for breaking into subject specific subgroups for discussion
Please RSVP here: https://www.airbnb.com/meetups/daywndmbd-airflow
We’re planning on starting to have Airflow meetups regularly, so stay tuned!
0 Comments